Crowdsourcing research can balance discussions, validate findings and better inform policy, say Raphael Silberzahn and Eric L. Uhlmann.
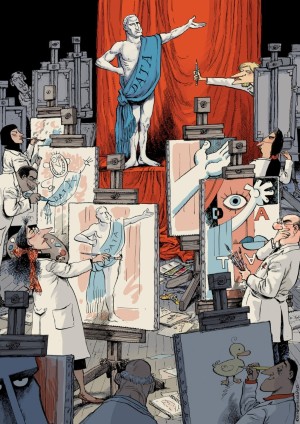
Our experience with crowdsourced analysis began in 2013, shortly after we published research1 suggesting that noble-sounding German surnames, such as König (king) and Fürst (prince), could boost careers. Another psychologist, Uri Simonsohn at the University of Pennsylvania in Philadelphia, asked for our data set. He was sceptical that the meaning of a person's name could affect life outcomes. While our results were featured in newspapers around the world, we awaited Simonsohn's response.
Re-running our analysis yielded the same outcome. But Simonsohn's different (and better) analytical approach showed no connection between a surname such as Kaiser (emperor) and a job in management. Despite our public statements in the media weeks earlier, we had to acknowledge that Simonsohn's technique showing no effect was more accurate. To make this finding public, we wrote a commentary with Simonsohn, in which we contrasted our analytical approaches and presented our joint conclusion2.
In analyses run by a single team, researchers take on multiple roles: as inventors who create ideas and hypotheses; as optimistic analysts who scrutinize the data in search of confirmation; and as devil's advocates who try different approaches to reveal flaws in the findings. The very team that invested time and effort in confirmation should subsequently try to make their hard-sought discovery disappear.
We propose an alternative set-up, in which the part of the devil's advocate is played by other research teams.
The experiment
Last year, we recruited 29 teams of researchers and asked them to answer the same research question with the same data set. Teams approached the data with a wide array of analytical techniques, and obtained highly varied results. Next, we organized rounds of peer feedback, technique refinement and joint discussion to see whether the initial variety could be channelled into a joint conclusion. We found that the overall group consensus was much more tentative than would be expected from a single-team analysis3.
The experience convinced us that bringing together many teams of skilled researchers can balance discussions, validate scientific findings and better inform policymakers. Here, we describe how such a crowdsourcing approach can be a useful addition to research.
In many academic disciplines, multiple teams work with the same data set, for instance the World Values Survey data in political science or genome databases in genetics research. However, each team is typically keen to investigate its own questions and search for new phenomena. Thus hypotheses and results are often held close. Only after a conclusion is ready for presentation are methods and outcomes shared with other researchers, leaving limited opportunity for critical discussion.
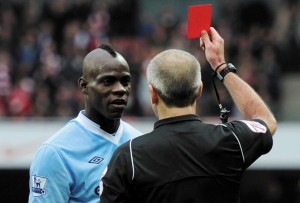
By contrast, our project set out to enable researchers to exchange methods and refine analyses before forming their conclusions. We asked the teams to approach the same data with the same question: are football (soccer) referees more likely to give red cards to players with dark skin than to players with light skin? This question touches on broad issues, such as how prejudice affects sports and how well the effects of prejudice, as detected in laboratory settings, show up in the real world.
Together with psychologist Brian Nosek, director of the Center for Open Science in Charlottesville, Virginia, and Dan Martin, a graduate student in quantitative psychology at the University of Virginia in Charlottesville, we developed a crowdsourcing methodology to coordinate analysts' efforts. Researchers who signed up for the project held varied opinions about whether an effect existed.
All teams were given the same large data set collected by a sports-statistics firm across four major football leagues. It included referee calls, counts of how often referees encountered each player, and player demographics including team position, height and weight. It also included a rating of players' skin colour. As in most such studies, this ranking was performed manually: two independent coders sorted photographs of players into five categories ranging from 'very light' to 'very dark' skin tone.
The teams independently tested their hypotheses. Each made its own decisions about how to best analyse the data set. We then took an inventory of all the approaches. Each team provided details such as which statistical model they used — everything from Bayesian clustering to logistic regression and linear modelling — what variables they used and why. Teams' approaches were then anonymized and sent back to all of the researchers without revealing results.
The researchers were asked to rate the validity of each approach and to provide in-depth feedback on three approaches. Then we sent participants a document listing all the approaches and associated feedback, and gave teams time to update their analyses. Then the groups documented their analyses and models, which, along with the results, were shared with all teams.
After that, we invited all the researchers to discuss the results through e-mail exchanges. Some approaches were deemed less defensible than others, but no consensus emerged on a single, best approach. After the discussion, we gave researchers the chance to add a note to their individual reports in light of others' work (in other words, to express doubts or confidence about their approach). Finally, we presented the teams' findings in a draft manuscript, which the participants were invited to comment on and modify.
Diversity of results
Of the 29 teams, 20 found a statistically significant correlation between skin colour and red cards (see 'One data set, many analysts'). The median result was that dark-skinned players were 1.3 times more likely than light-skinned players to receive red cards. But findings varied enormously, from a slight (and non-significant) tendency for referees to give more red cards to light-skinned players to a strong trend of giving more red cards to dark-skinned players. After reviewing each other's reports, most team leaders concluded that a correlation between a player having darker skin and the tendency to be given a red card was present in the data.
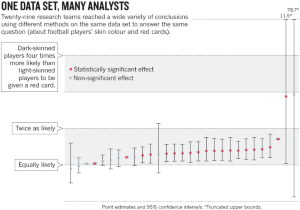
Nonetheless, the fact that so many analytical approaches can be presented — and justified — gives researchers and the public a more nuanced view. Any single team's results are strongly influenced by subjective choices during the analysis phase. Had any one of these 29 analyses come out as a single peer-reviewed publication, the conclusion could have ranged from no race bias in referee decisions to a huge bias.
Most researchers would find this broad range of effect sizes disturbing. It means that taking any single analysis too seriously could be a mistake, yet this is encouraged by our current system of scientific publishing and media coverage.
Pros and cons
For many research problems, crowdsourcing analyses will not be the optimal solution. It demands a huge amount of resources for just one research question. Some questions will not benefit from a crowd of analysts: researchers' approaches will be much more similar for simple data sets and research designs than for large and complex ones. Importantly, crowdsourcing does not eliminate all bias. Decisions must still be made about what hypotheses to test, from where to get suitable data, and importantly, which variables can or cannot be collected. (For instance, we did not consider whether a particular player's skin tone was lighter or darker than that of most of the other players on his team.) Finally, researchers may continue to disagree about findings, which makes it challenging to present a manuscript with a clear conclusion. It can also be puzzling: the investment of more resources can lead to less-clear outcomes.
Under the current system, strong storylines win out over messy results.
Still, the effort can be well worth it. Crowdsourcing research can reveal how conclusions are contingent on analytical choices. Furthermore, the crowdsourcing framework also provides researchers with a safe space in which they can vet analytical approaches, explore doubts and get a second, third or fourth opinion. Discussions about analytical approaches happen before committing to a particular strategy. In our project, the teams were essentially peer reviewing each other's work before even settling on their own analyses. And we found that researchers did change their minds through the course of analysis.
Crowdsourcing also reduces the incentive for flashy results. A single-team project may be published only if it finds significant effects; participants in crowdsourced projects can contribute even with null findings. A range of scientific possibilities are revealed, the results are more credible and analytical choices that seem to sway conclusions can point research in fruitful directions. What is more, analysts learn from each other, and the creativity required to construct analytical methodologies can be better appreciated by the research community and the public.
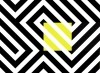
Of course, researchers who painstakingly collect a data set may not want to share it with others. But greater certainty comes from having an independent check. A coordinated effort boosts incentives for multiple analyses and perspectives in a way that simply making data available post-publication does not.
The transparency resulting from a crowdsourced approach should be particularly beneficial when important policy issues are at stake. The uncertainty of scientific conclusions about, for example, the effects of the minimum wage on unemployment, and the consequences of economic austerity policies should be investigated by crowds of researchers rather than left to single teams of analysts.
Under the current system, strong storylines win out over messy results. Worse, once a finding has been published in a journal, it becomes difficult to challenge. Ideas become entrenched too quickly, and uprooting them is more disruptive than it ought to be. The crowdsourcing approach gives space to dissenting opinions.
Scientists around the world are hungry for more-reliable ways to discover knowledge and eager to forge new kinds of collaborations to do so. Our first project had a budget of zero, and we attracted scores of fellow scientists with two tweets and a Facebook post.
Researchers who are interested in starting or participating in collaborative crowdsourcing projects can access resources available online. We have publicly shared all our materials and survey templates, and the Center for Open Science has just launched ManyLab, a web space where researchers can join crowdsourced projects.
References
-
Silberzahn, R. & Uhlmann, E. L. Psychol. Sci. 24, 2437–2444 (2013).
-
Silberzahn, R., Simonsohn, U. & Uhlmann, E. L. Psychol. Sci. 25, 1504–1505 (2014).
-
Silberzahn, R. et al. Preprint available at https://osf.io/j5v8f (2015).
Author information
Authors and Affiliations
Corresponding authors
Related links
Related links
Related links in Nature Research
Blind analysis: Hide results to seek the truth 2015-Oct-07
Robust research: Institutions must do their part for reproducibility 2015-Sep-01
Over half of psychology studies fail reproducibility test 2015-Aug-27
First results from psychology’s largest reproducibility test 2015-Apr-30
Scientific method: Statistical errors 2014-Feb-12
Reproducibility: The risks of the replication drive 2013-Nov-20
Weak statistical standards implicated in scientific irreproducibility 2013-Nov-11
Rights and permissions
About this article
Cite this article
Silberzahn, R., Uhlmann, E. Crowdsourced research: Many hands make tight work. Nature 526, 189–191 (2015). https://doi.org/10.1038/526189a
-
Published
-
Issue Date
-
DOIhttps://doi.org/10.1038/526189a